The Role of Artificial Intelligence and Machine Learning in Asteroid Detection
Blogs Home
- 04 Jul 2023
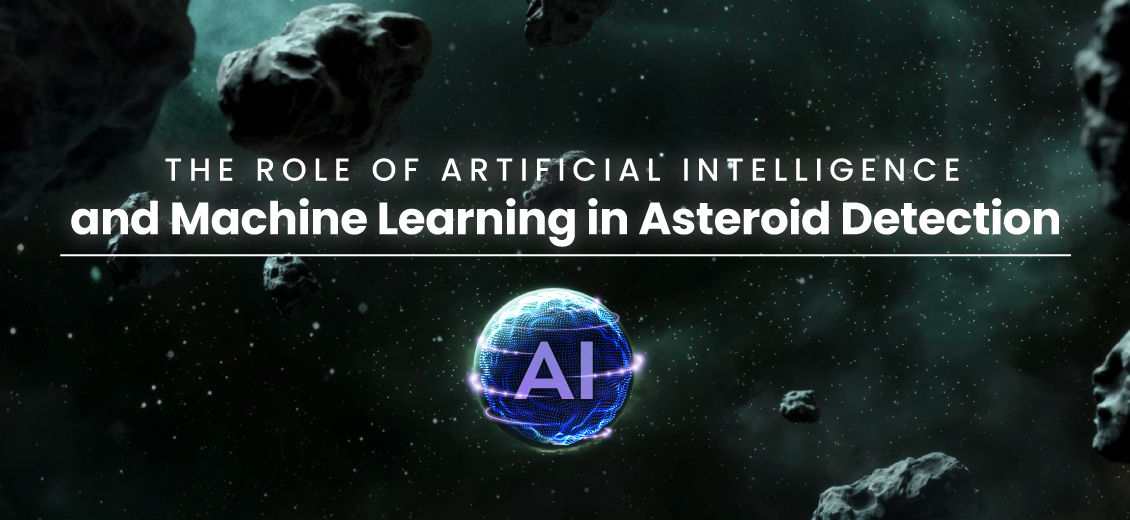
At a UN conference, a dinosaur appears out of the blue and takes the dias amongst a scared and bewildered elite audience urging the delegates from different nations not to choose extension by subsidising fossil fuels and heading towards a climate disaster; "at least we had an asteroid" it highlights. This is a climate action campaign by UNDP. When I hear the word asteroid, the Chicxulub event is the first thing that comes to my mind. Nearly 66 million years ago, an approximate 10 km asteroid hit the Gulf of Mexico wiping out 75% of existing life on Earth including dinosaurs (widely known as Cretaceous-Tertiary or K-T mass extinction event); inducing sudden changes across all ecosystems. The impact of the event makes the need for study and research on asteroid detection and related technologies self-explanatory.
Asteroids
Asteroids are the rocky bodies (mostly irregular in shape) orbiting the Sun that were formed as our solar system originated. They are neither as large and spherical as dwarf planets nor have air or gas-like comets. They range from as large as a thousand km wide to as small as a few meters and have craters from collisions with other asteroids. Some of them have moons while others revolve around each other in a pair or three asteroid systems. They have been categorised as per their composition into c-type (carbon-rich and most common), s-type (silicon-rich) and m-type (metallic: iron and nickel-rich). Based on their location they may be classified into Asteroid belt, Trojans and Near Earth Asteroids (NEA).
Importance of Studying Asteroids
The composition of asteroids is similar to that of inner planets but they have remained largely unaffected by factors that have changed rocks on Earth, Moon or Mars. Hence, they are the best sources to understand the origin of our solar system and planets. There are chances that water and life were brought to Earth by asteroids which makes them crucial to understanding the genesis of life. Mineral-rich composition of asteroids is a profitable mining target aiding further space exploration and 'colonisation'. At some point in future, they may serve as fuel and water sources for astronauts. Despite their lucrativeness, they are a potential impact hazard to life on Earth.
Asteroid Impact Hazards
Astrohazards can have a devastating impact on the Earth. NEA collide with Earth on a daily basis, countered simply by the atmosphere, appearing as beautiful shooting stars; history, however, has more to tell. The 30th of June marked as International Asteroid Day by the UN to increase awareness of asteroid impact hazards, which happened to be the anniversary of the largest recorded asteroid impact in modern history, the Tunguska event (Siberia, Russia) that released the energy equivalent to 185 Hiroshima bombs. The Chicxulub mass extinction is probably the most famous impact event. The most recent one was in 2018 after the Chelyabinsk (Russia) event in 2013. There are over 30, 000 NEA which pose an impact threat to the Earth requiring dedicated study, discovery, identification (with regard to potential impact) and mitigation measures. In order to avoid loss of property, human, plant, animal life or ecosystem or a major change in the biosphere that may catalyse mass extinction, early asteroid detection becomes necessary.
Traditional Approaches for Asteroid Detection
Earliest asteroid detections began with manual charting of moving objects with handmade sketches of several positions. Then came ground-based telescopic photography, utilizing stereoscopes. It was followed by CCD-based telescopes. Radar use for asteroid detection began early around 1968, which allowed asteroid characterization as well. Conventionally using telescopic imagery an expert astronaut would generate a 3D model of an asteroid based on simple computer programs for shape modelling, which could take anywhere between 1 to 3 months. These are time-consuming, require more human resources and are less efficient for real-time monitoring of threats. Ground-based telescopes are usable only during the night and are highly dependent on the clarity of skies (including haze, cloud and light pollution). In recent decades, astronomical data collection technology has improved significantly, releasing huge volumes of data for processing. This has called for capitalising on the robust ubiquitous technology of Artificial Intelligence (AI) for the early detection of asteroids.
AI: It refers to the science of developing programmes and machines that are capable of 'intelligence' (the ability to think and act rationally) to facilitate problem-solving and decision-making. AI may be classified as narrow or strong, where the former stands for specific task-oriented programs like Alexa while the latter is yet a theoretical concept with two sub-categories: General AI which shall possess an intelligence level equivalent to that of humans and Superintelligence AI where the intelligence level will surpass that of humans.
Machine Learning (ML): It is a branch of AI where using a given data set and algorithms a machine learns to perform a task and improve its accuracy by iterating the algorithm. It consists of a 'decision process' to classify by observing the data patterns; an 'error function' to estimate model accuracy and 'model optimisation' entailing iterations of the decision process to adjust data weights until the desired accuracy is reached.
Applications of AI and ML:
- Speech recognition: Siri, Alexa etc.
- Recommendation engines: Personalised recommendations like on YouTube or online shopping apps like Myntra
- Automated customer service: Virtual customer assistants and chatbots
- Computer vision: Facial recognition, MRI analysis, self-driven cars
- Fraud detection; automated stock trading.
These abilities make them further applicable in nearly every discipline.
AI and ML in Asteroid Detection
Advanced technology is accredited with delivering colossal data sets for asteroid detection. But the amount of data comes with its own challenges for processing, making manual detection all the more tedious and AI and ML-based asteroid extraction all the more necessary. Before any algorithms can be applied to the imagery obtained from optical telescopes, space telescopes and radar preprocessing are required for raw data. It usually includes registration, background noise removal, filtering and data reduction; however, in some ML programmes, these processes may be inbuilt. Softwares like NEARBY, PANGU or other ML softwares like R, Javascript or Python-based suites allow the processing of these datasets. The algorithms focus on moving object detection as compared to the rather stationary background of stars.
Sources of Data and Processing
- Ground-Based Telescopes (GBT): Catalina Sky Survey (CSS) (in Arizona) PanSTARRS, ATLAS, Schmidt-Cassegrain telescope by NASA, Very Large Telescope (ESA), and other state and privately owned telescopes.
- Space Telescopes: Hubble Space Telescope (the first space telescope! NASA-ESA), James-Webb Space Telescope(ESA, NASA, CSA), NEO WISE (NASA), AKIRRA (JAXA), ASTROSAT(ISRO)
- Radar Telescopes: Green Bank Telescope (West Virginia, NASA), ngRADAR, Deep Space Network, Arecibo Observatory, Goldstone Deep Space Communications, RT-70, Pluton (Russia). While the telescopes provide imagery, radar data is captured as echo reflection of the radio waves projected to the source; echo power distribution in time delay and Doppler frequency produces images with better special resolution. Unlike GBT radar works during the daytime, in clouds and dust; they are also good at locating objects in a given time and space.
Processing of data includes pre-processing (registration, noise removal using existing libraries and image segmentation) and further application of appropriate algorithms on the preprocessed image to extract asteroids and thereafter accuracy assessment. Large-scale and complex datasets are challenging to handle: full of noise, time-consuming, requiring enormous storage capacity and robust models that do not slow down during processing. Highly human-dependent processing may consume a lot of resources and lead to delays in timely detection.
AI and ML Techniques in Asteroid Detection
The machine learning algorithms to detect asteroids fall mainly into 3 categories which involve the classification of the image data to predict the different number of groups emerging in the data:
- Supervised Classification: When a labelled training data set is provided to the computer to learn and use on the rest of imagery to classify it and extract features. Frequently used algorithms in this category for asteroids are decision trees, random forest, logistic regression and support vector machines. The decision tree contains nodes and based on the answer yes or no tree is split further. Random forest uses multiple decision trees.
- Unsupervised Classification: Involves algorithm processing on unlabeled data in which it identifies patterns and iterates itself to provide output classes until threshold accuracy is reached. The top relevant algorithms in this category are clustering, artificial neural networks and anomaly detection. Clustering is best suited for large unlabeled data where outputs are the classes formed by grouping the closest pixels together. Anomaly detection helps in identifying outliers, changes in events or drifts. Artificial neural networks process data by mimicking the human brain like nodes or artificial neurons. Nodes and edges have weights associated with that change as the algorithm trains itself; neural networks involve a series of algorithms.
- Semi-Supervised Classification: Employs a small labelled data set for large unlabeled data to train the algorithm.
Convolution Neural Networks (CNN), a neural algorithm that has a convolution layer reducing data dimensionality, assigns weights to input layer objects and differentiates between different objects. This along with ANN and is being used increasingly to deal with large astronomical data sets and maybe supervised or semi-supervised.
Case Studies and Success Stories
- Asteroid Institute together with Google cloud computing identified 104 asteroids using a new algorithm called THOR (Tracklet-less Heliocentric Orbit Recovery)
- DeepAsteroid is a software model using the machine learning platform Tensorflow (Google's open-source deep learning platform) that was in NASA’s top 25 space apps challenge award.
- NASA asteroid data hunter allows people across the world to upload images from their telescopes and load them for detection.
- Neoaid was jointly developed by Aerospace AI engineers and Nvidia engineers at the CSS facility.
Future Prospects
ESA and NASA are heading into several interesting asteroid detection projects that shell include detailed identification of asteroids which may eventually lead to their use as space stations, water sources or mining purposes. Test-bed is an autonomous ground-based telescope programme under development by ESA, with two telescope locations in Spain and Australia. The first of ESA's FLY-EYE ground telescopes using a compound insect eye concept to provide finer details will be installed in Sicily in 2023. NEO Surveyor is a space-based telescope mission planned by NASA. NASA is also planning a mission LUCY to study Trojan asteroids DART was a successful asteroid deflection project by NASA. HERA by ESA will monitor the impact of DART on the smaller asteroid it deflected from its position. Hera will have on board decision making and automation that will reduce the amount of data sent to Earth limiting it to meaningful information.
Challenges
One of the concerning challenges with using ML for asteroid detection is the quality of data. Model results are limited by what quality of data entered. Also, models tend to overfit or underfit. Algorithms may have an inherent bias based on how they have been trained which sometimes limits their flexibility. They are trained on a limited dataset in labs and may not completely depict or capture the real-world phenomenon, here asteroids. Deep learning-based ANN and CNN often require huge data which means robust systems and GPU to process it as well as high power consumption. The increasing space programs and observatories increase the space debris; as some of these become unoperational they present a threat to International Space Station as well as Earth. AI and ML also come with challenges of transparency and reliability; often the model preparation may be rushed for demonstrating the successful application. AI and ML have a long-existing problem of the 'black-box problem': the inability to explain how the result was arrived at. Then there are ethical considerations as AI has been increasingly found to infringe data privacy Excessive advancement of AI in any field poses the ultimate threat of surpassing human control and hence needs proper regulation through laws.
Conclusion
Asteroid early detection and warning are essential as they are the hazards that have changed the course of history. Scientific advancement calls for the application of AI and ML in asteroid hunting. But AI and ML can not detect each and every asteroid out there. All existing systems must be used in coordination and under human supervision. They pose a great opportunity to use the vast volumes of astronomical data along with their traditional drawbacks of bias, lack of transparency and flexibility. This calls for developing more robust ML modules to process the data keeping a human-in-loop, SOPs at institution levels and stringent laws governing AI applications. Under guided SOPs, AI and ML will usher in the era of early and automated asteroid detection in the coming times.
References:
Harris, A.W. (2011). Asteroid. In: , et al. Encyclopedia of Astrobiology. Springer, Berlin, Heidelberg.
https://doi.org/10.1007/978-3-642-11274-4_116
https://www.esa.int/Space_Safety/About_asteroids_and_Planetary_Defence
https://lish.harvard.edu/asteroid-data-hunter-challenge
https://www.un.org/en/observances/asteroid-day
https://www.lpi.usra.edu/features/asteroids/110512/
https://www.jpl.nasa.gov/edu/news/2017/4/18/how-nasa-studies-and-tracks-asteroids-near-and-far/
Adam, Coralie D. “DART’s Data Verify Its Smashing Success at Deflecting Asteroid Moon Dimorphos.” DART’s Data Verify Its Smashing Success at Deflecting Asteroid Moon Dimorphos, 19 Apr. 2023, www.nature.com/articles/d41586-023-01020-1.
https://www.lpi.usra.edu/education/explore/solar_system/background/background4.shtml
https://www.un.org/en/observances/asteroid-day
https://www.ibm.com/topics/machine-learning
“Machine Learning and Next-generation Asteroid Surveys.” NASA/ADS, ui.adsabs.harvard.edu/abs/2017DPS....4910303N/abstract. Accessed 25 June 2023.
Carruba, V., Aljbaae, S., Domingos, R.C. et al. Machine learning applied to asteroid dynamics. Celest Mech Dyn Astron 134, 36 (2022).
Hefele, John D., et al. “Identifying Earth-impacting Asteroids Using an Artificial Neural Network | Astronomy and Astrophysics (a&A).” Identifying Earth-impacting Asteroids Using an Artificial Neural Network | Astronomy & Astrophysics (a&A), 4 Feb. 2020,
www.aanda.org/articles/aa/full_html/2020/02/aa35983-19/aa35983-19.html.
https://doi.org/10.1007/s10569-022-10088-2
“The Daily Processing of Asteroid Observations by Gaia.” The Daily Processing of Asteroid Observations by Gaia - ScienceDirect, 27 Nov. 2015,
www.sciencedirect.com/science/article/abs/pii/S003206331500358X.
Meech, K., Weryk, R., Micheli, M. et al. A brief visit from a red and extremely elongated interstellar asteroid. Nature 552, 378–381 (2017). https://doi.org/10.1038/nature25020
V. Bahel, P. Bhongade, J. Sharma, S. Shukla and M. Gaikwad, "Supervised Classification for Analysis and Detection of Potentially Hazardous Asteroid," 2021 International Conference on Computational Intelligence and Computing Applications (ICCICA), Nagpur, India, 2021, pp. 1-4, doi: 10.1109/ICCICA52458.2021.9697222.
https://www.aanda.org/articles/aa/full_html/2022/05/aa42998-21/aa42998-21.html
https://ieeexplore.ieee.org/abstract/document/6850925
“Towards Asteroid Detection in Microlensing Surveys With Deep Learning.” - ScienceDirect, 30 Jan. 2023, www.sciencedirect.com/science/article/pii/S2213133723000082.
https://adsabs.harvard.edu/full/1992acm..proc..541S
Sakshi Naithani
Sakshi has done her Masters in Geography from Delhi School of Economics, University of Delhi. She is currently pursuing Ph.D. in natural hazard risk assessment from Indian Institute of Remote Sensing-Kumaun University. When not engaged in research work, she loves to write poetry.
Blogs Home