Machine Learning and Artificial Intelligence
Blogs Home
- 21 Jan 2023
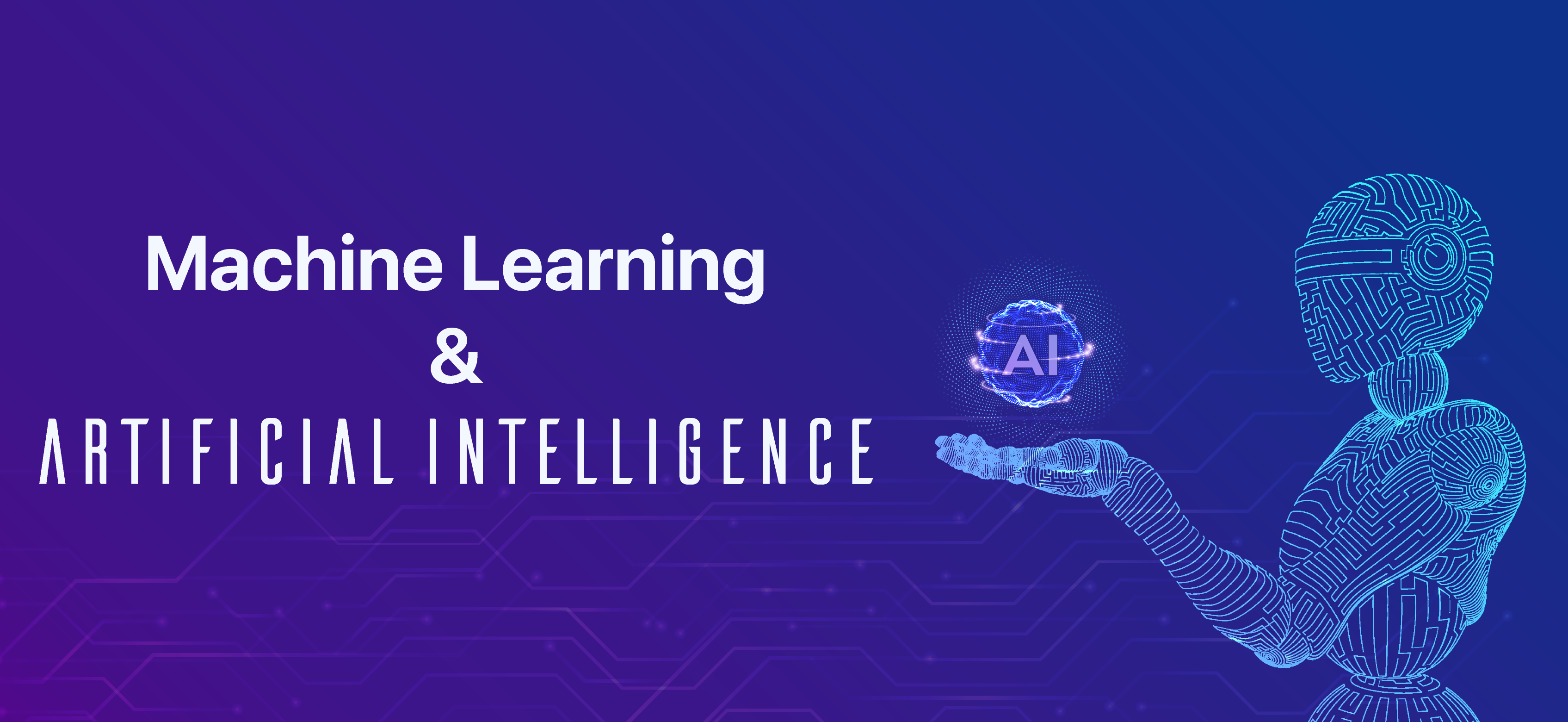
Machine learning and artificial intelligence are two of the most exciting and rapidly advancing fields in technology today. Both have the potential to transform a wide range of industries, from healthcare and finance to transportation and manufacturing. In this blog post, we will explore what these technologies are, how they work, and some of the key applications and challenges associated with them.
Machine learning is a method of teaching computers to learn from data, without being explicitly programmed. It involves using algorithms to analyze and draw insights from data, and then using those insights to make predictions or decisions. There are three main types of machine learning: supervised learning, unsupervised learning, and reinforcement learning.
Supervised learning is the most common type of machine learning. In this method, the computer is given a set of labeled data, where the desired output is already known. The computer then uses this data to train a model, which can be used to make predictions on new, unseen data. For example, a supervised learning algorithm could be used to train a model to identify whether an image contains a cat or a dog.
Unsupervised learning, on the other hand, involves working with unlabeled data. The computer is not given any information about the desired output, and must instead find patterns and structure in the data on its own. This type of learning is often used for tasks such as clustering, where the computer groups similar data points together, or dimensionality reduction, where the computer finds a lower-dimensional representation of the data.
Reinforcement learning is a type of machine learning that involves training a model to make decisions or take actions in an environment in order to achieve a desired outcome. This type of learning is often used for tasks such as robotics, where the machine must learn to navigate and interact with its environment.
Artificial intelligence, or AI, is a broader term that encompasses both machine learning and other techniques for building intelligent systems. At its core, AI is the simulation of human intelligence in machines. It encompasses a wide range of techniques, including natural language processing, computer vision, and decision-making.
Applications of Machine Learning and AI
One of the key applications of machine learning and AI is in the field of healthcare. These technologies have the potential to revolutionize the way we diagnose and treat disease. For example, machine learning algorithms can be used to analyze medical images and make accurate diagnoses of conditions such as cancer. Additionally, AI-powered virtual assistants can help patients manage their health by providing personalized health information and tracking their symptoms.
Another important application of machine learning and AI is in the field of finance. These technologies can be used to analyze large amounts of financial data and make predictions about market trends and the performance of individual stocks. This can be used to help traders make more informed trades. This technology is also being used to detect and prevent fraud, as well as to make better investment decisions.
One of the most popular applications of ML is in computer vision. This is the use of algorithms to analyze and understand images and videos. In the manufacturing industry, computer vision is used to inspect products and detect defects.
Another important application of ML is Natural Language Processing (NLP). This is the use of algorithms to understand and generate human language. NLP is being used in a variety of applications, such as chatbots and virtual assistants, which can understand and respond to human speech and text. This technology is also being used to analyze and understand large volumes of text data, such as customer reviews or social media posts, to gain insights and make better decisions.
AI is also being used in the field of robotics and automation. Robotics is the use of machines to perform tasks that would be difficult or dangerous for humans. With the help of AI, robots are becoming more intelligent and are able to perform a wider range of tasks. This technology is being used in manufacturing, transportation, and logistics to improve efficiency and reduce costs.
In the field of transportation, AI and ML are being used to develop self-driving cars, which can navigate roads and make decisions without human input. This technology is also being used to optimize traffic flow, reduce congestion, improve public transportation, and even manage air traffic control.
Finally, AI and ML are also being used to improve energy efficiency, by optimizing the use of renewable energy sources, such as solar and wind power.
Overall, the applications of AI and ML are diverse and far-reaching, and this technology is expected to play an increasingly important role in our daily lives in the coming years. As the technology continues to evolve, it will open up new possibilities and opportunities, and change the way we live and work in the future.
Challenges Encountered
Despite the many benefits of machine learning and AI, there are also some important challenges that need to be addressed. One of the biggest challenges is ensuring that these technologies are fair and unbiased. Because machine learning algorithms are trained on large amounts of data, they can inadvertently perpetuate the biases that are present in that data. This can lead to unfair or discriminatory outcomes.
One major challenge is the availability of high-quality data. Machine learning algorithms rely on large amounts of data to learn and make predictions. However, obtaining high-quality data can be difficult and time-consuming. Data may be incomplete, inconsistent, or biased, which can negatively impact the performance of the machine learning model. Additionally, the data may not be representative of the real-world environment in which the model will be used, which can lead to poor performance when the model is deployed.
A further challenge is the complexity of the models themselves. As machine learning models become more advanced, they can become increasingly complex and difficult to understand. This can make it difficult for practitioners to interpret the results of a model and make decisions based on those results. Additionally, complex models can be more difficult to debug and optimize, making it challenging to improve the model's performance.
Another challenge is the ability of the model to generalize to new data. A model that is trained on a specific set of data may not perform well when presented with new, unseen data. This is known as overfitting, and it can be difficult to address without additional data or modifications to the model.
There are also ethical and societal challenges to consider. As artificial intelligence becomes more sophisticated, there are concerns about its impact on privacy and accountability. For example, as more data is collected and analyzed, there is a risk of privacy violations and the misuse of data. Ensuring that artificial intelligence is developed and used in a responsible and ethical manner is crucial to its long-term success.
Another challenge is interpretability, as machine learning models are often considered as "black boxes" which it is hard to understand how they make their predictions. This can make it difficult to trust the results of the model, particularly in sensitive areas such as medical diagnosis or credit risk assessment.
Finally, there is the question of job displacement. As machines become better at performing certain tasks, there is a concern that they will replace human workers. This is particularly true in industries where repetitive and manual tasks are common, such as manufacturing and transportation. However, it's important to note that these technologies also have the potential to create new jobs and increase productivity.
It's also important to consider that machine learning and AI can augment human capabilities, rather than replace them. For example, AI-assisted medical diagnosis can help doctors make more accurate diagnoses, which can lead to better patient outcomes. Similarly, self-driving cars can reduce the number of accidents caused by human error, making our roads safer for everyone.
In conclusion, machine learning and artificial intelligence are powerful technologies that have the potential to revolutionize a wide range of industries. While there are some important challenges that need to be addressed, which includes bias and interpretability, the availability of high-quality data, the complexity of the models, the ability of the model to generalize to new data, ethical and societal challenges, interpretability and the ability to deploy models in real-world environments. These technologies also have the potential to improve our lives in countless ways. It's important that we continue to invest in research and development in these fields, to ensure that we can fully realize their potential and address any negative impacts that may arise.
Aditi Saini
After pursuing Master's in Political Science from Indraprastha College for Women, Delhi University, she engrossed herself in Content Writing. Apart from being honest and a kind person, she is a good learner with the motto of "Live and let live".
Blogs Home